Voting: A machine learning approach
The journal article was published by Dávid Burka (Department of Computer Science), Clemens Puppe (Karlsruhe Institute of Technology), László Szepesváry (Department of Operations Research and Actuarial Sciences) and Attila Tasnádi (Department of Mathematics) in the European Journal of Operational Research (Aip: 85).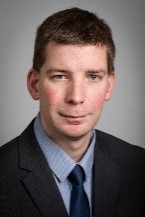
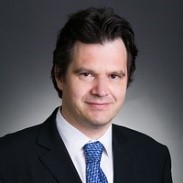
Voting rules can be assessed from quite different perspectives: the axiomatic, the pragmatic, in terms of computational or conceptual simplicity, susceptibility to manipulation, and many others aspects. In this paper, we take the machine learning perspective and ask how ‘well’ a few prominent voting rules can be learned by a neural network. To address this question, we train the neural network to choosing Condorcet, Borda, and plurality winners, respectively. Remarkably, our statistical results show that, when trained on a limited (but still reasonably large) sample, the neural network mimics most closely the Borda rule, no matter on which rule it was previously trained. The main overall conclusion is that the necessary training sample size for a neural network varies significantly with the voting rule, and we rank a number of popular voting rules in terms of the sample size required.
Link: https://authors.elsevier.com/c/1e3Gz_8axWgn3o