Exploration of the investment patterns of potential retail banking customers using two-stage cluster analysis – Publication by Tibor Kovács, Andrea Kő and Asefeh Asemi
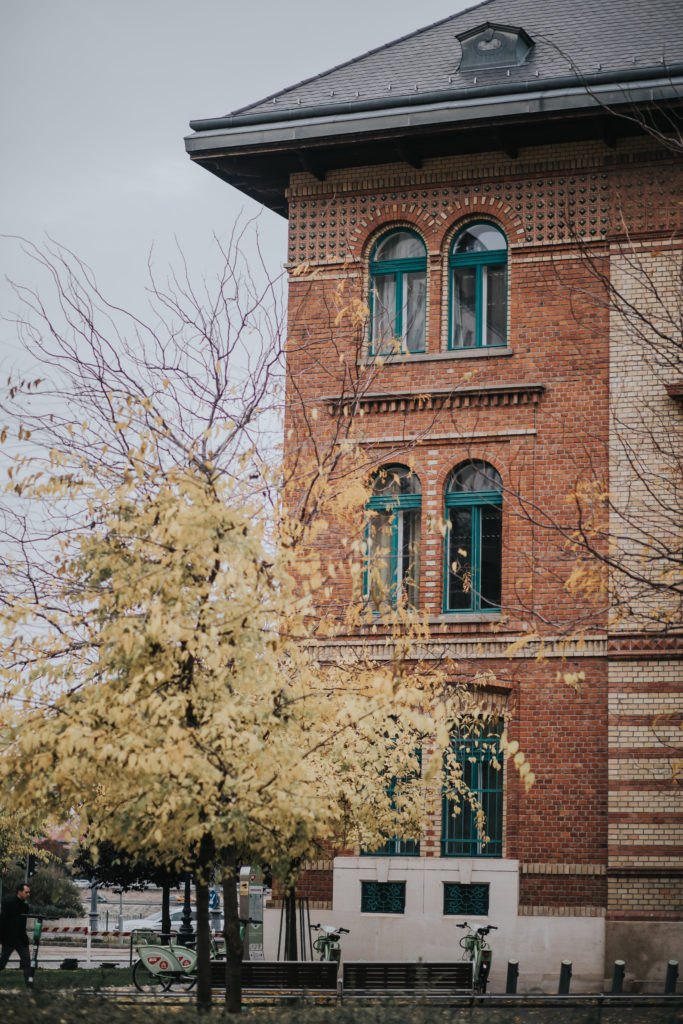
Identifying investment patterns as part of customer segmentation is one of the most important tasks in retail banking. Clustering customers effectively is an important element of improving marketing policy and strategic planning. There are several methods for identifying similar groups of customers and describing their characteristics to offer them appropriate products. However, using machine learning methods is rare, and the application is limited for certain types of data. The aim of this study is to investigate the benefits of using a two-stage clustering method using neural-network-based Kohonen self-organizing maps followed by hierarchical clustering for identifying the investment patterns of potential retail banking customers. The unique benefit of this method is the ability to use both categorical and numerical variables at the same time. This research examined 1,542 responses received for an online investment survey, focusing on the questions that are related to the respondents’ investment preferences and their current financial assets. The research utilizes descriptive statistics and multiple correspondence analysis (MCA) to understand the variables and Kohonen self-organizing maps (SOMs), in combination with hierarchical clustering, to identify customer groups and describe the characteristics of these clusters. The analysis was able to identify clusters of potential customers with similar preferences and gained insights into their investment patterns related to their investment portfolio and investment behavior, including their savings profile, attitude to risk-taking, and preferences for investment advice. These findings were supported by additional insights through the application of multiple correspondence analysis (MCA) describing patterns of financial instruments and portfolios. The main contribution of the research is the combined application of the machine learning methods Kohonen SOM, hierarchical clustering, and MCA for investment pattern analysis in the retail banking business.
Kovacs, T., Ko, A., & Asemi, A. (2021). Exploration of the investment patterns of potential retail banking customers using two-stage cluster analysis. Journal of Big Data, 8(1), 141. https://doi.org/10.1186/s40537-021-00529-4
Related contacts
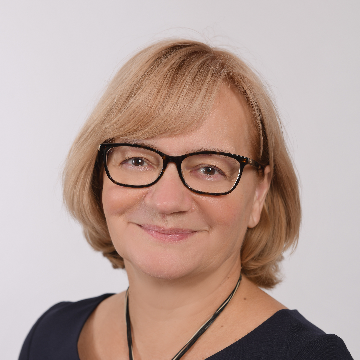
dr. Kő Andrea
Intézetvezető, Egyetemi tanár / Head of Institute, Professor
Rektori Szervezet / Adatelemzés és Informatika Intézet
Kovács Tibor
Egyetemi Docens / Associate Professor
Rektori Szervezet / Adatelemzés és Informatika Intézet / Információrendszerek Tanszék