Modern Methods of Conic Optimization – Yurii Nesterov’s minicourse
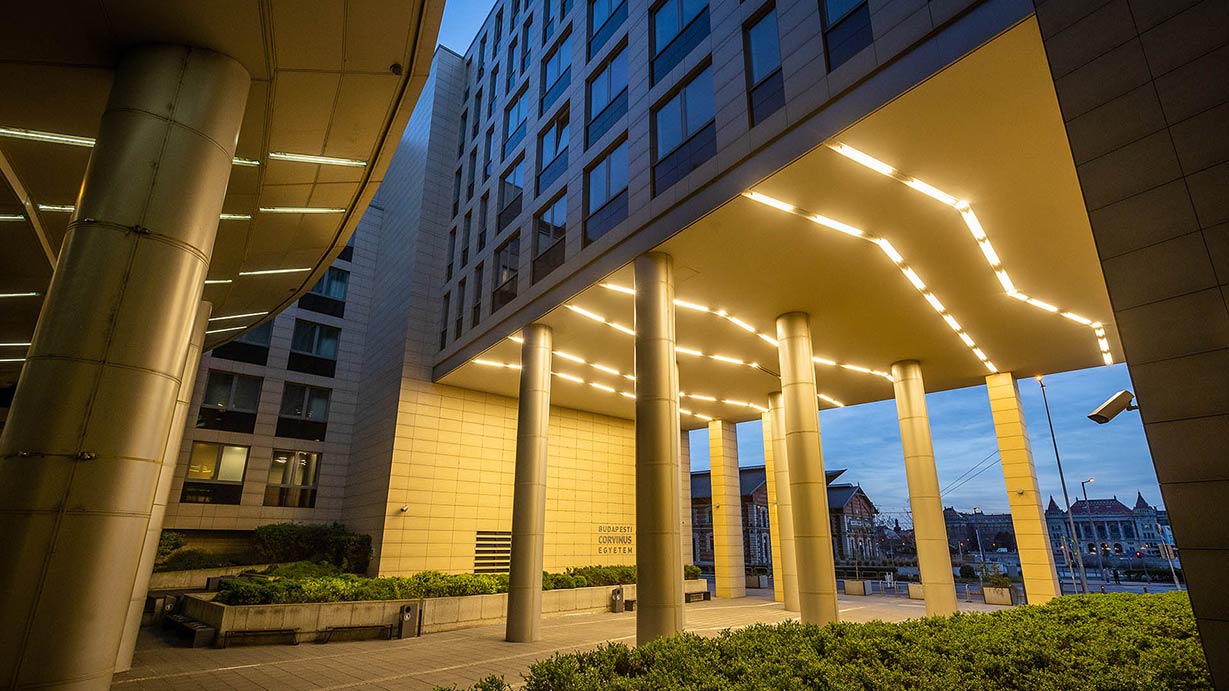
Date:
October 14. (Monday) 9:50-11:20 (Room E.3.340)
October 15. (Tuesday) 9:50-11:20 (Room C325)
October 15. (Tuesday) 13:40-15:10 (Room C315)
Venue: Corvinus University of Budapest, Building E and C
We would like to inform you that the lectures will be broadcast online. Please be aware that this broadcast will not utilize professional-grade equipment, and we appreciate your understanding that we cannot ensure the broadcast’s quality.
If you would like to join online, please send an e-mail to petra.rigo@uni-corvinus.hu by October 13.
Abstract
In this course, we present the most efficient primal-dual interior-point methods (IPMs) for solving Conic Optimization Problems over general convex cones. In the first lecture, we refresh the main facts from the theory of self-concordant functions, which are necessary for development of the primal-dual IPMs. We study the properties of the primal-dual central path and present the most efficient potential-reduction methods and long-step path-following schemes. Our analysis is based on a non-standard notion of the functional neighborhood of the central path.
In the second lecture, we present a homogenization approach, which eliminates the necessity of finding for IPM a feasible starting point in a close neighborhood of the central path. We discuss the abilities of the corresponding primal-dual methods in detecting different types of infeasibility, which can possibly occur in the pair of primal-dual problems.
In the last third lecture, we discuss our abilities in constructing non-symmetric primal-dual IPM. For the standard framework, we need to form computable primal-dual barriers for the primal and dual cones. If one of these barriers is difficult to compute, we need to modify our search strategies in order to keep the best polynomial-time complexity bounds and the long-step abilities of the methods.